Plan & Execute 최종회 - 이 소스코드와 DeepSeek R1 의 응답을 비교해 보았습니다. 결과는 ... ㄷㄷㄷ
2025. 2. 14. 08:27 |
이번 영상에서는 LangGraph의 Plan & Execute 추론을 다룬 소스코드 분석 마지막 편입니다.
소스코드 분석이지만 개발자가 아닌 분들도 보시면 재미 있으실 겁니다.
AI 분야에서 추론은 어떻게 진행되는지 한번 확인해 보세요.
사용자의 질문을 작은 Task들로 나누고, 이를 하나하나 단계적으로 실행하면서 **Re-plan(재계획)**하는 과정을 구현했습니다.
이러한 접근 방식 덕분에, 더 높은 수준의 정보를 사용자에게 제공할 수 있는 AI Agent를 만들 수 있습니다.
🎯 과연 이 방식이 ChatGPT, Claude, DeepSeek, Perplexity 같은 최신 AI 모델들과 비교했을 때 어떤 차이를 보일까요?
실험을 위해 **"가장 유명한 K-Pop 걸그룹의 각 멤버 생일을 알려줘"**라는 질문을 AI 모델들에게 던졌습니다:
비디오에서 이 소스코드가 답한 내용과 현재 가장 성능이 좋은 AI 모델들이 답한 내용을 비교해 보세요.
특히 DeepSeek R1 의 추론과 답은 놀랍더라구요.
https://youtu.be/dtyjji0Mgxo?si=1_SFwLZDKvTs5Htk
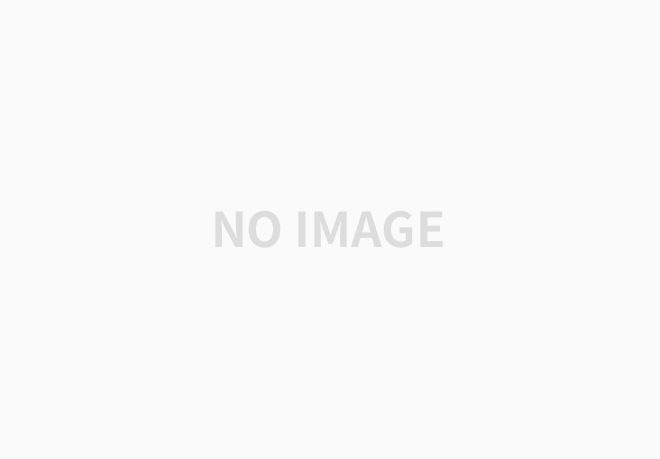
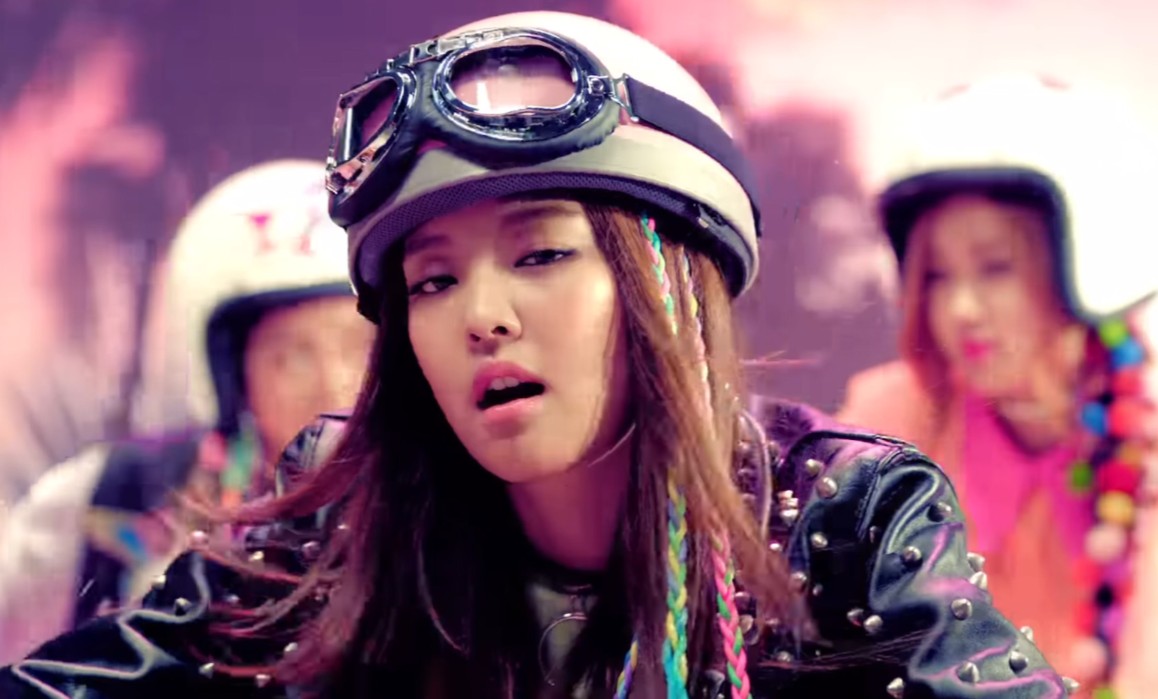
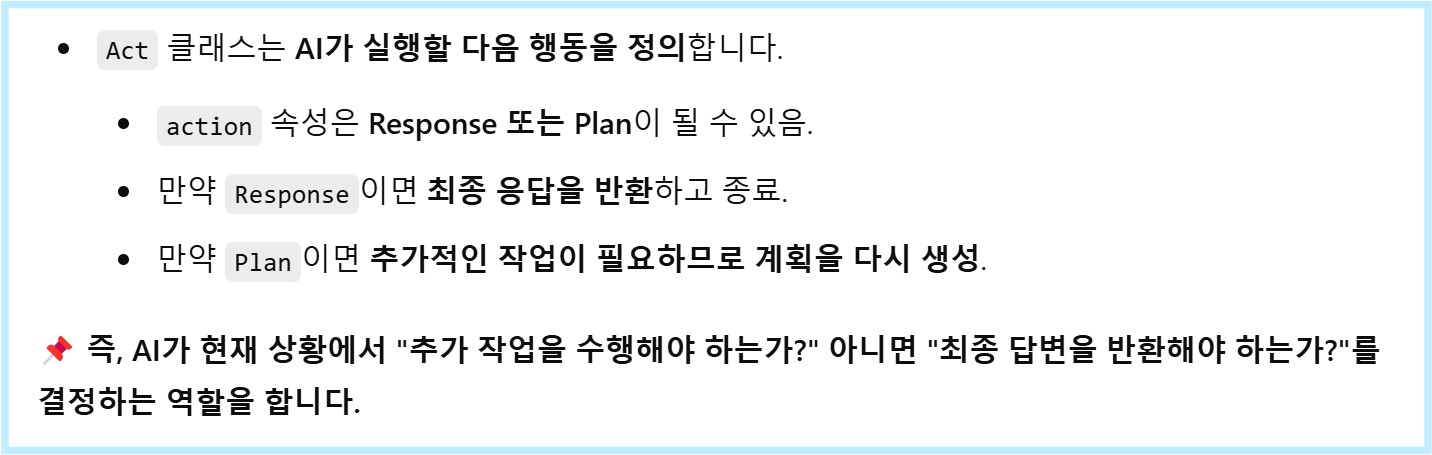
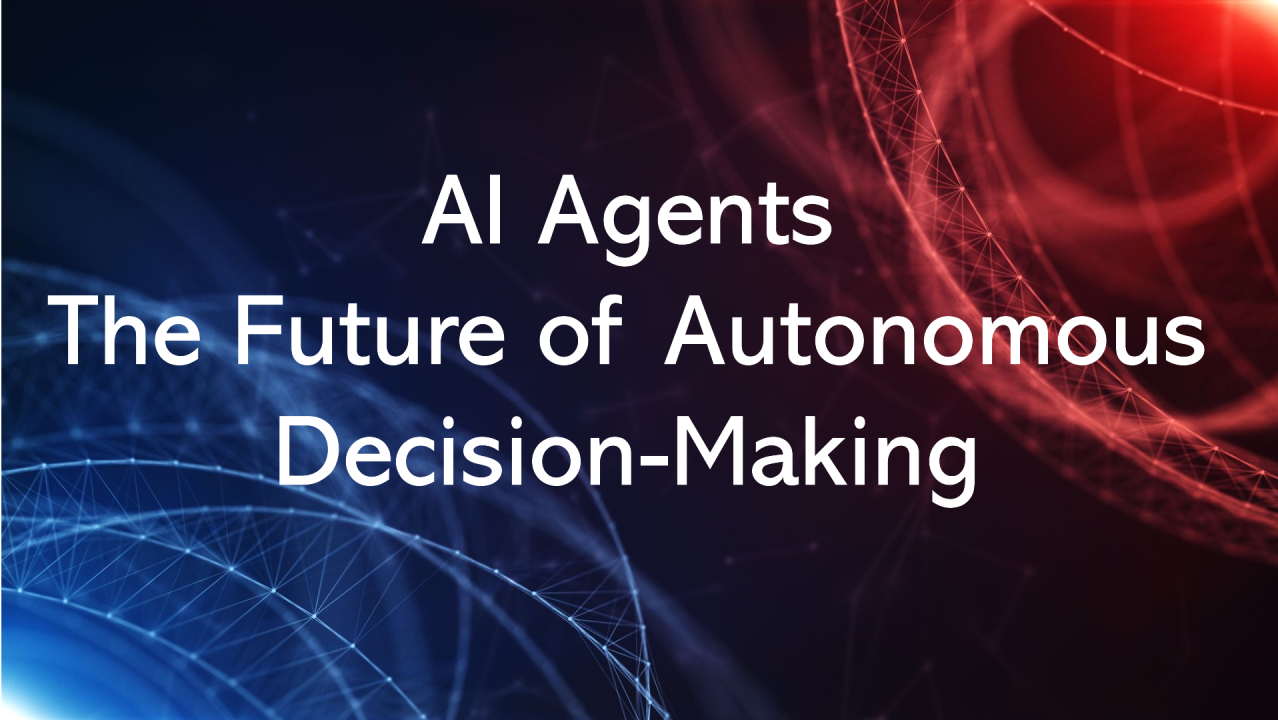
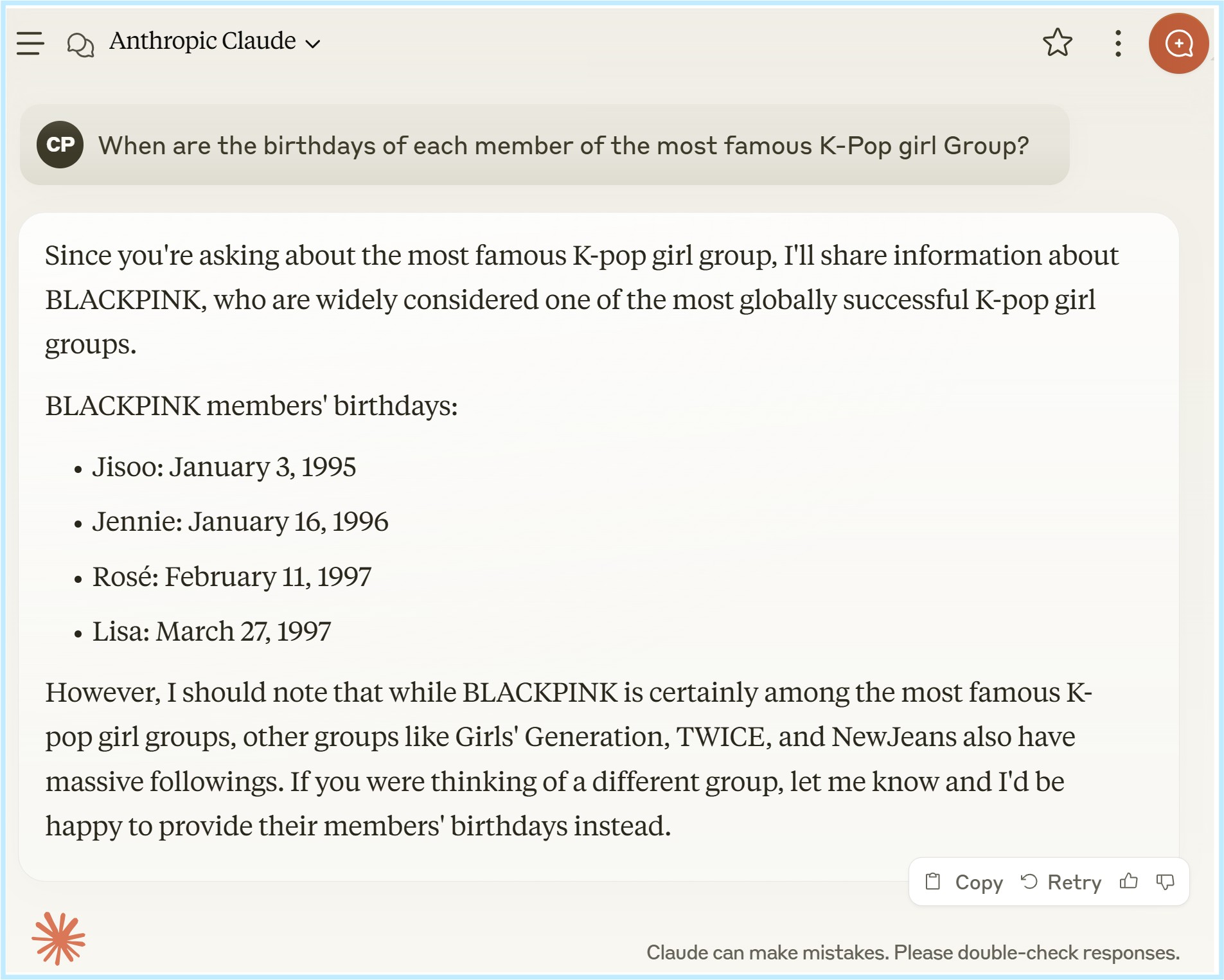
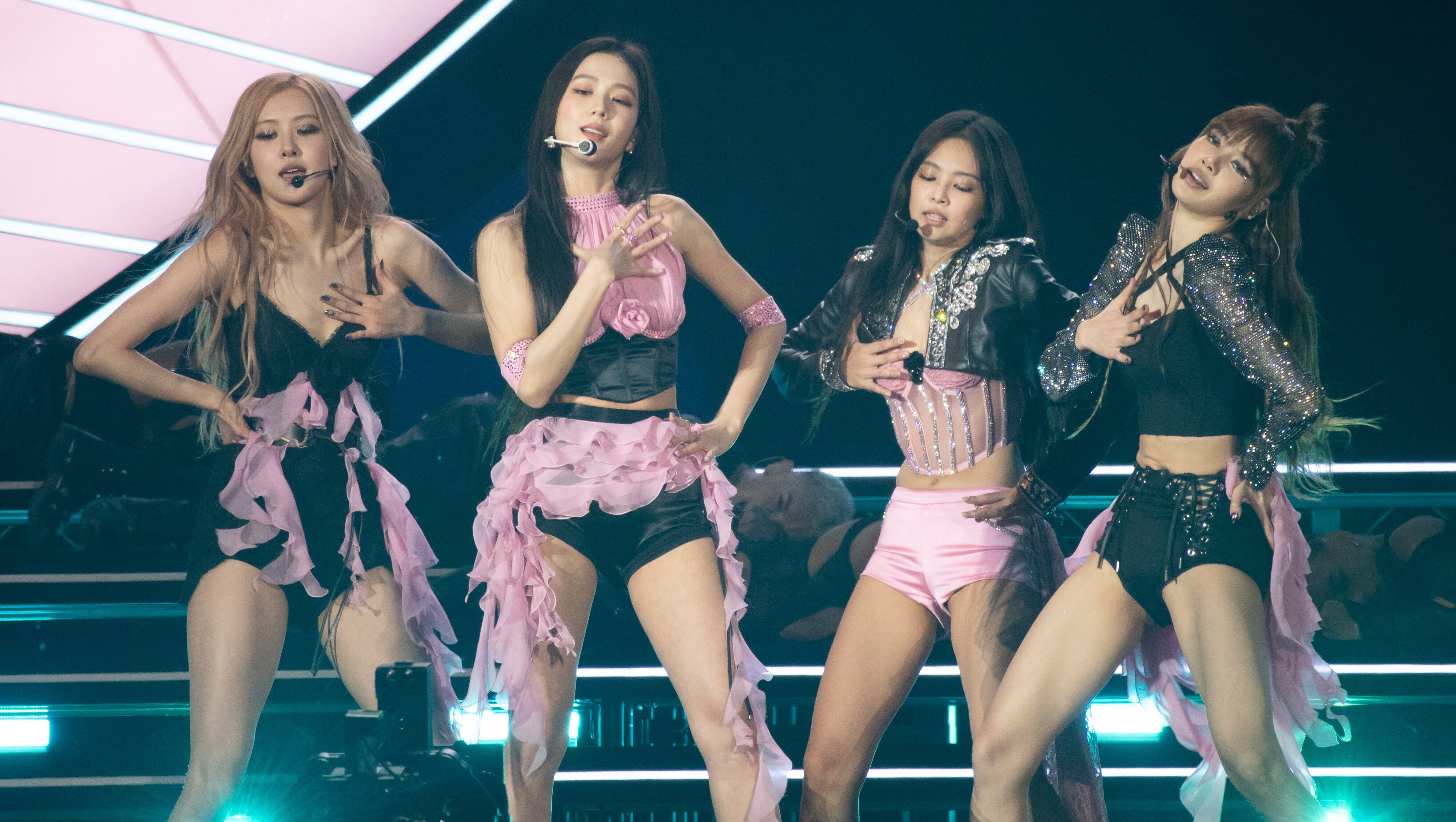
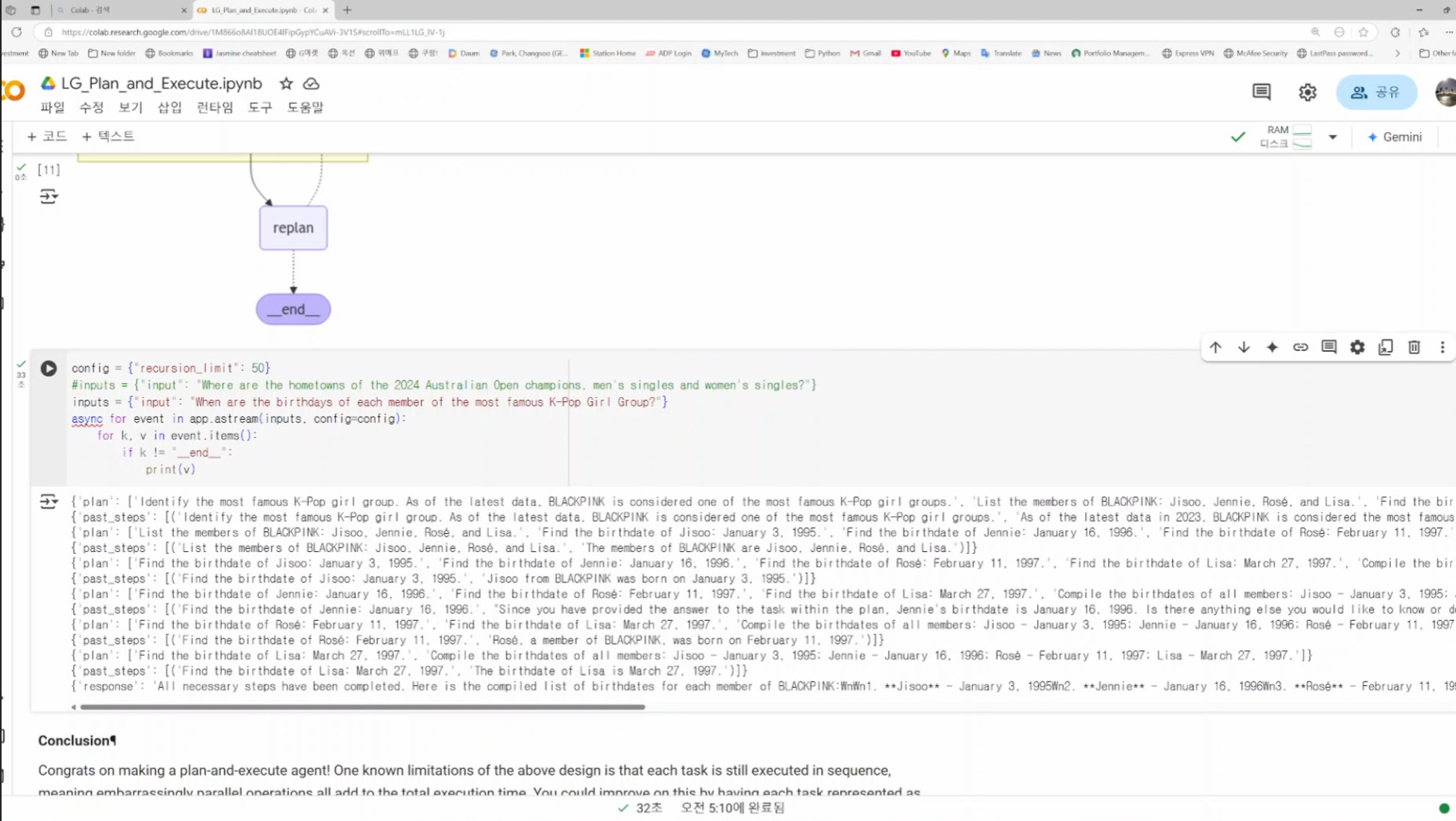
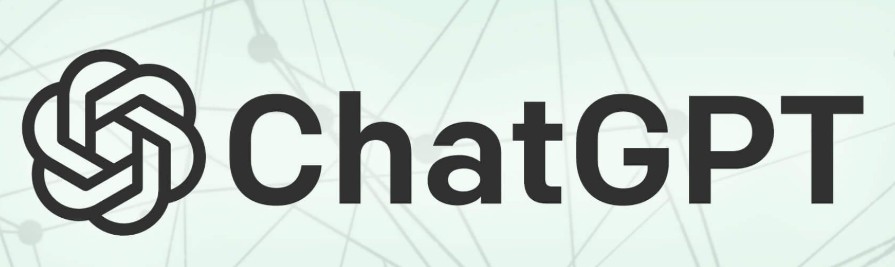
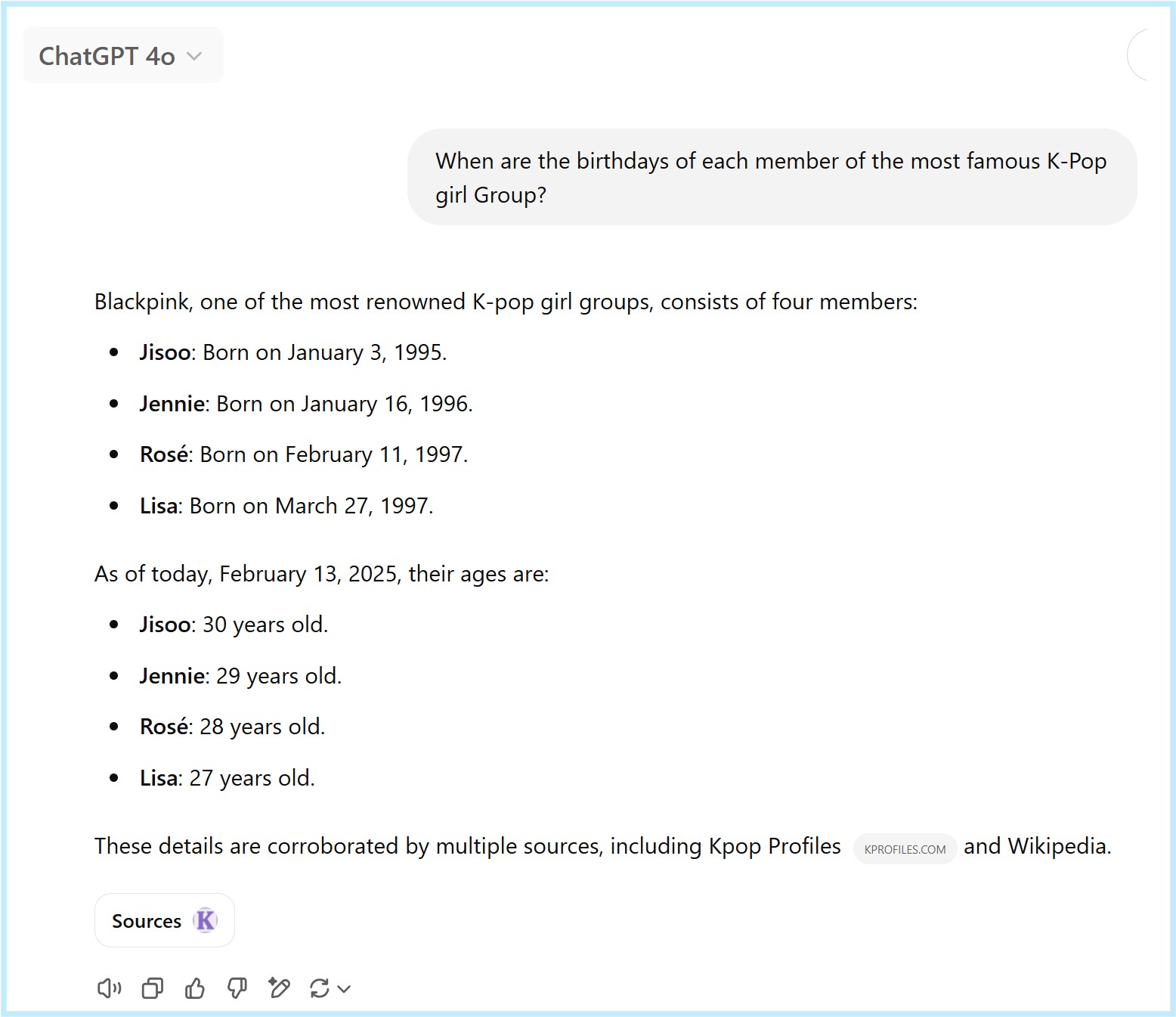
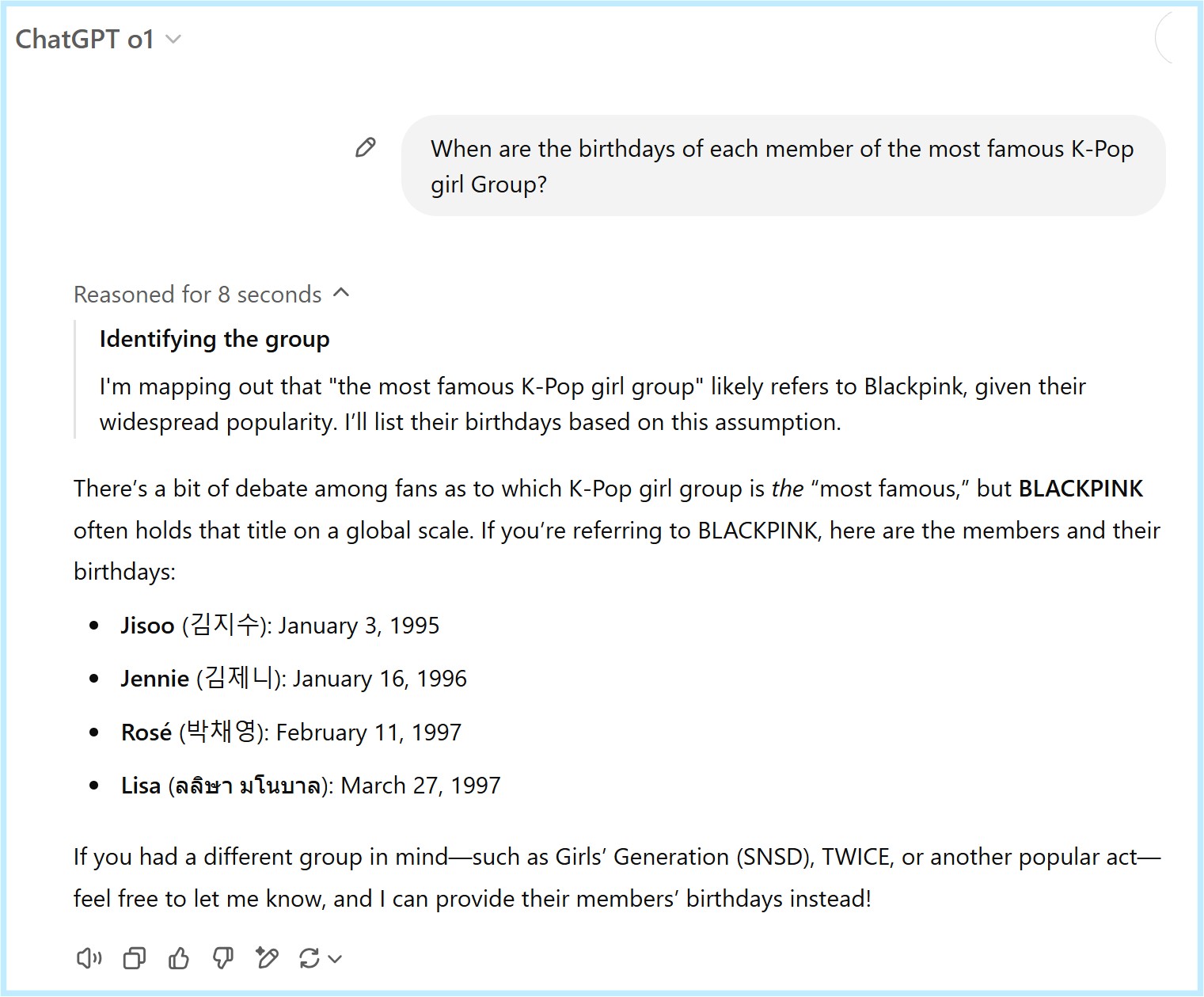
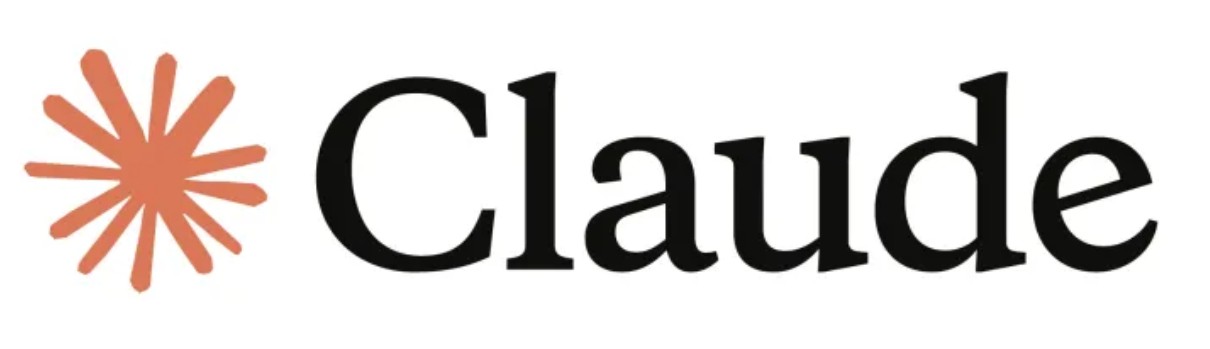
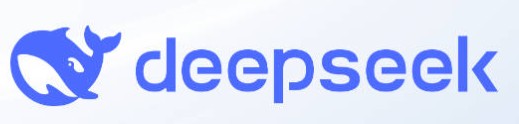
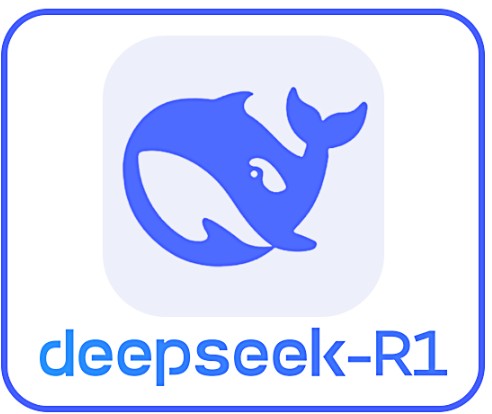
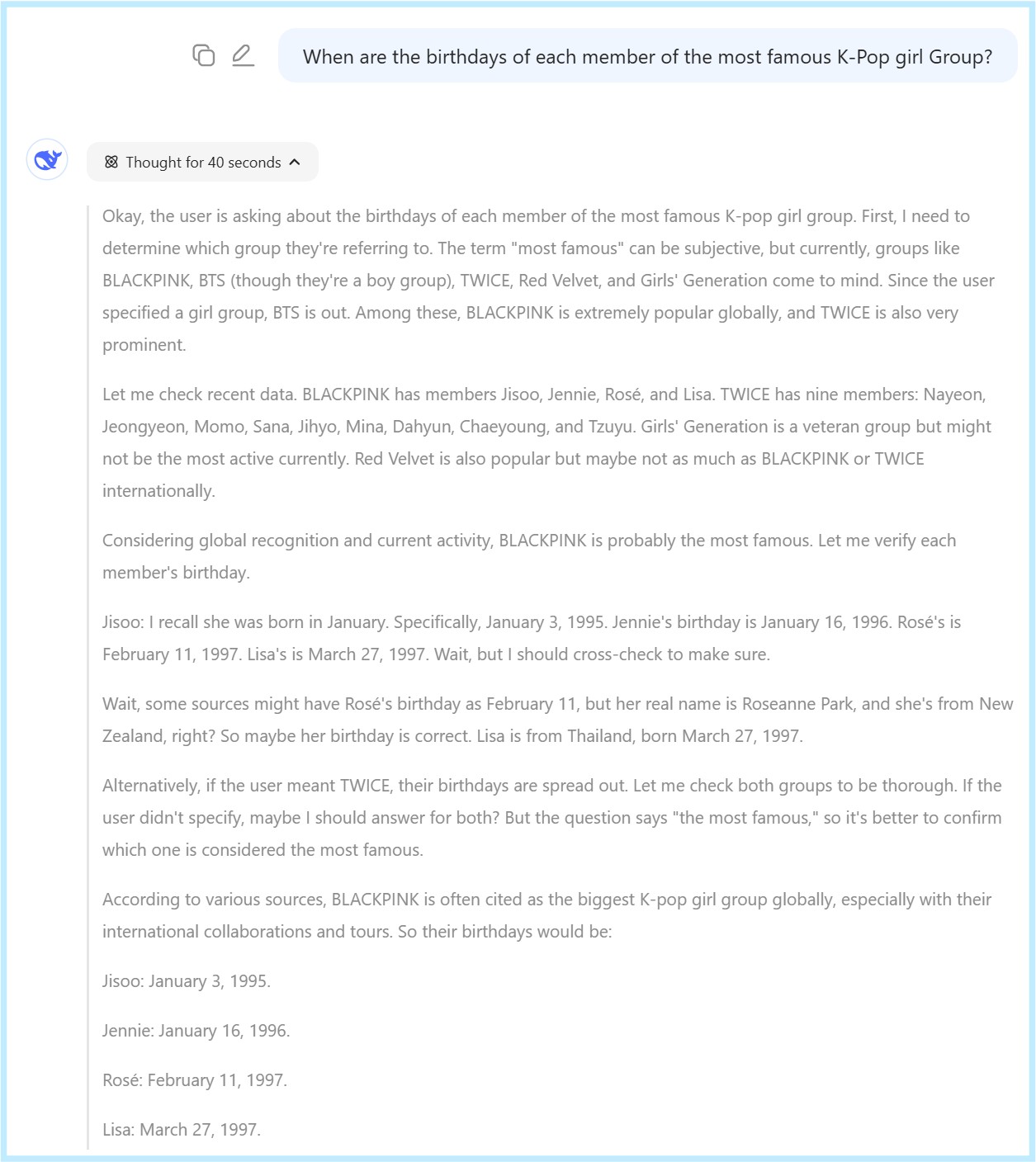
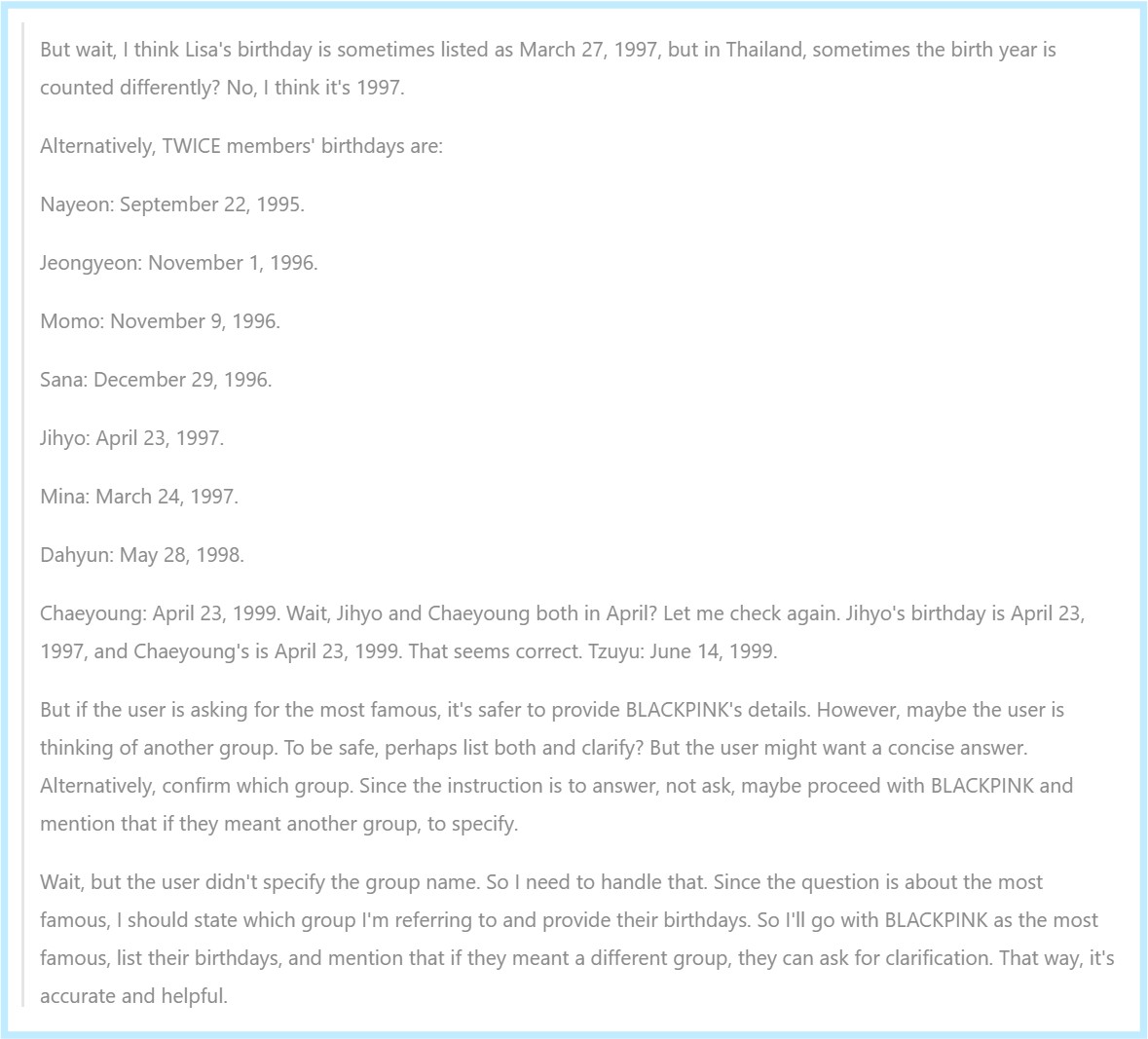
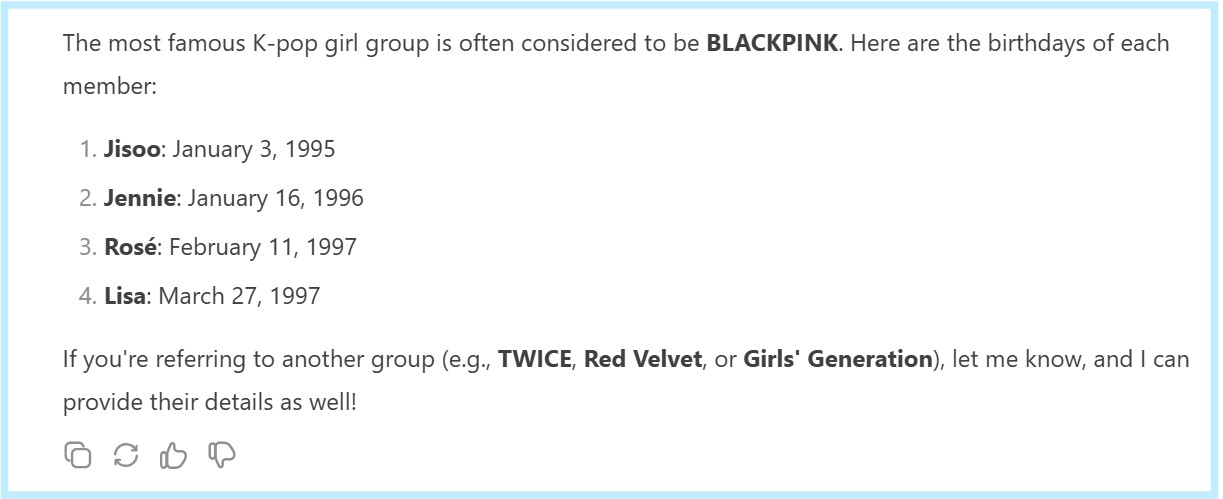
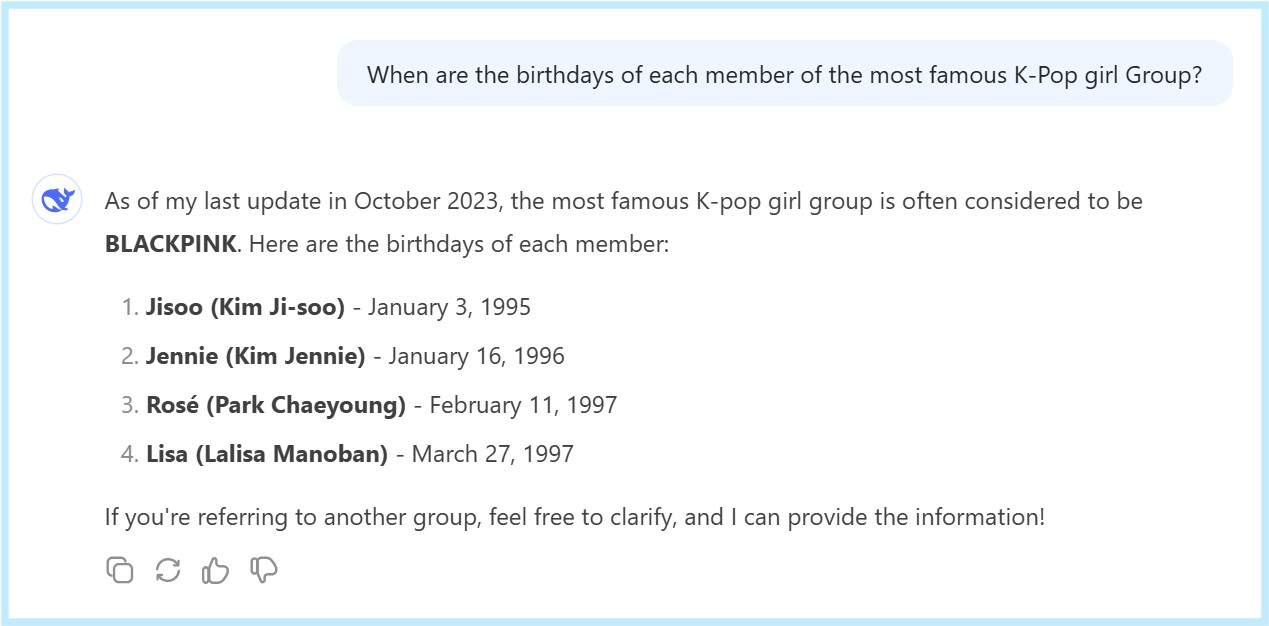
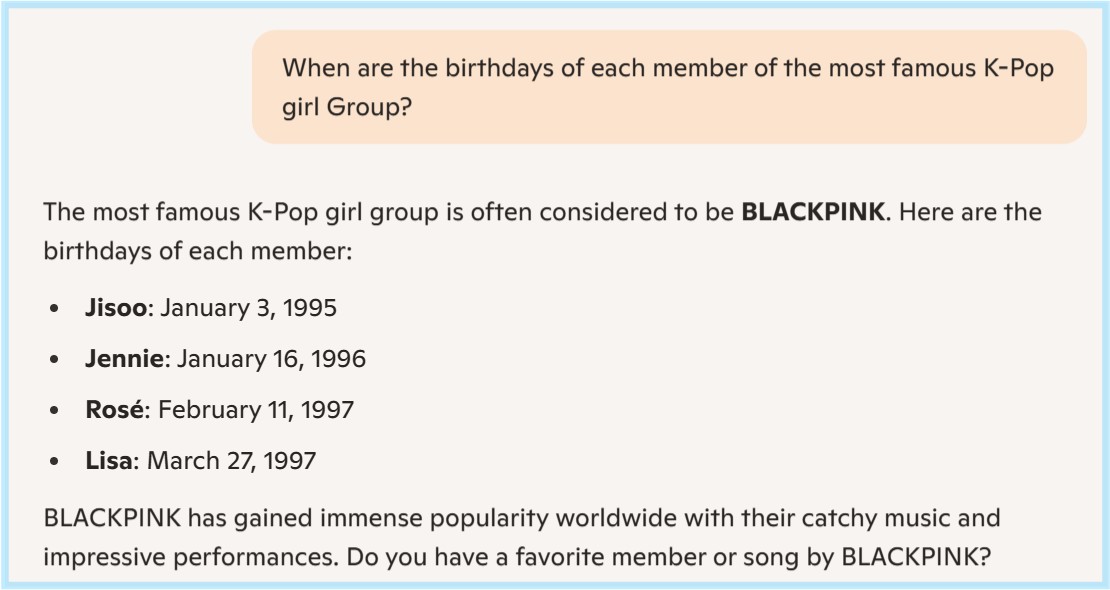
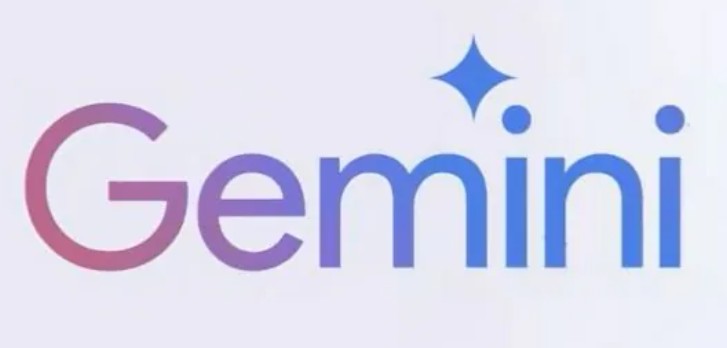
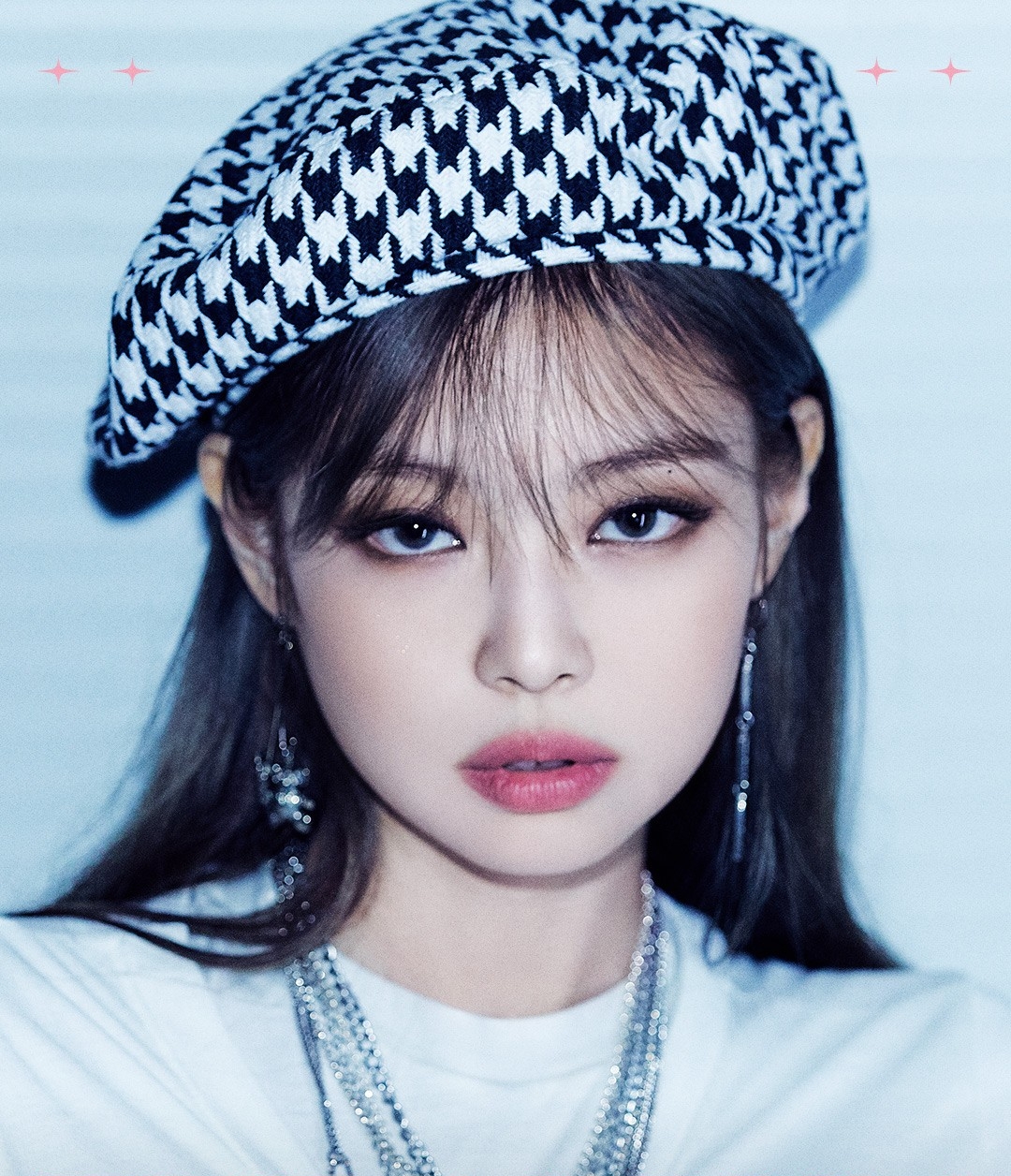
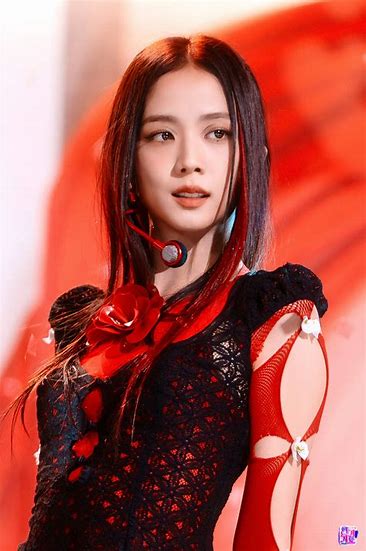
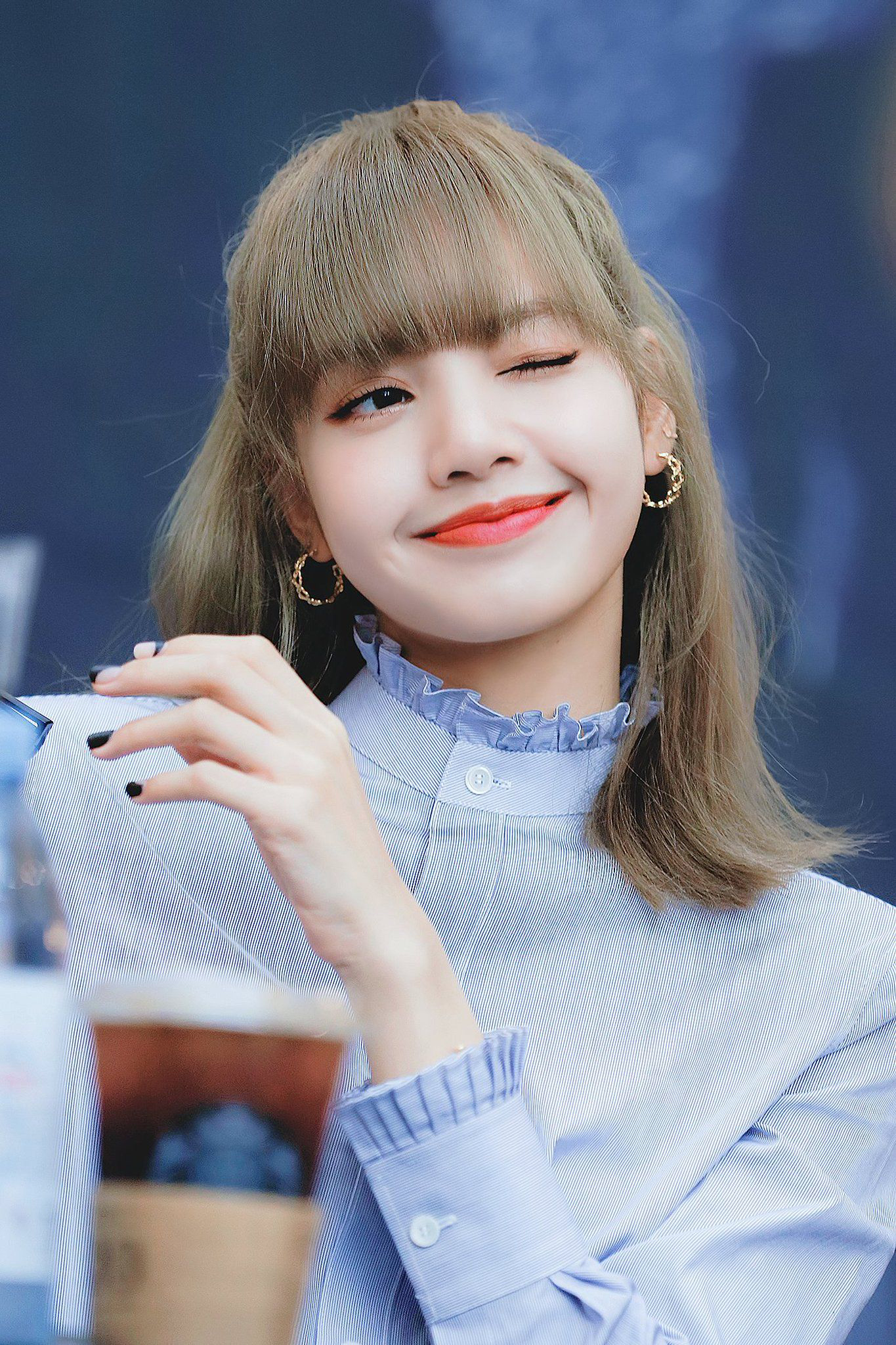
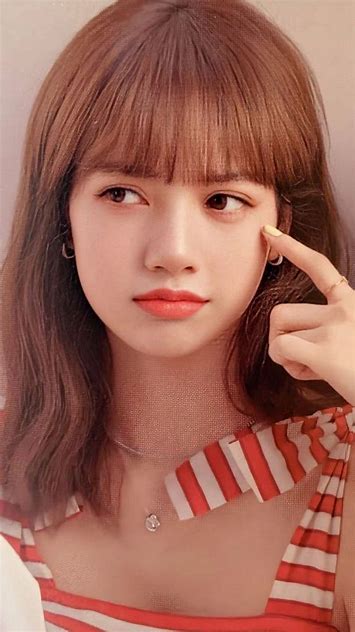
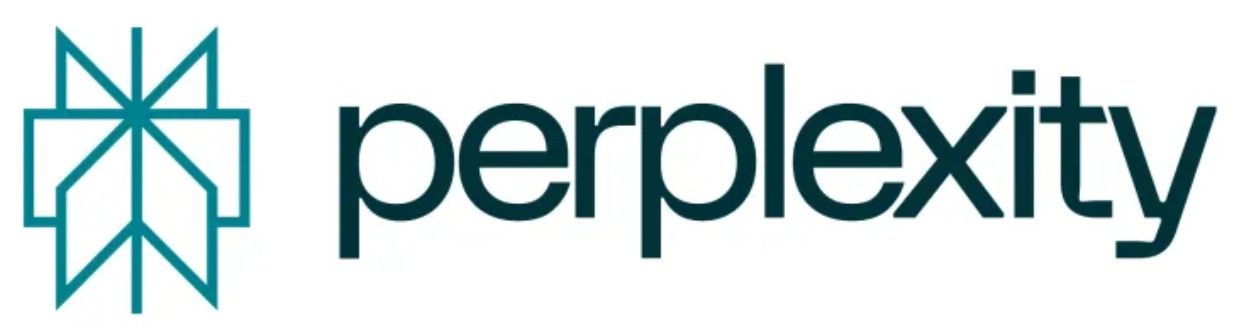
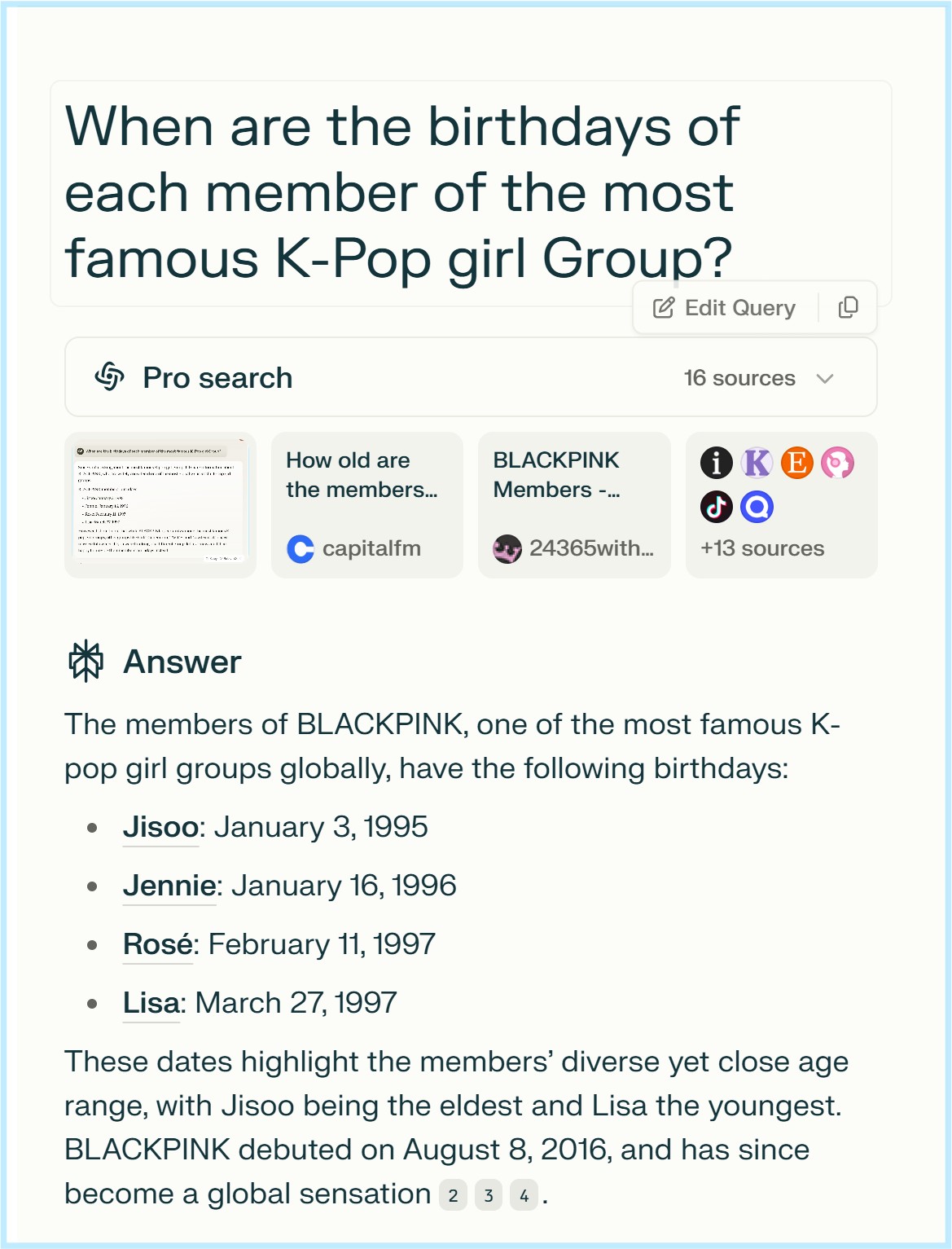

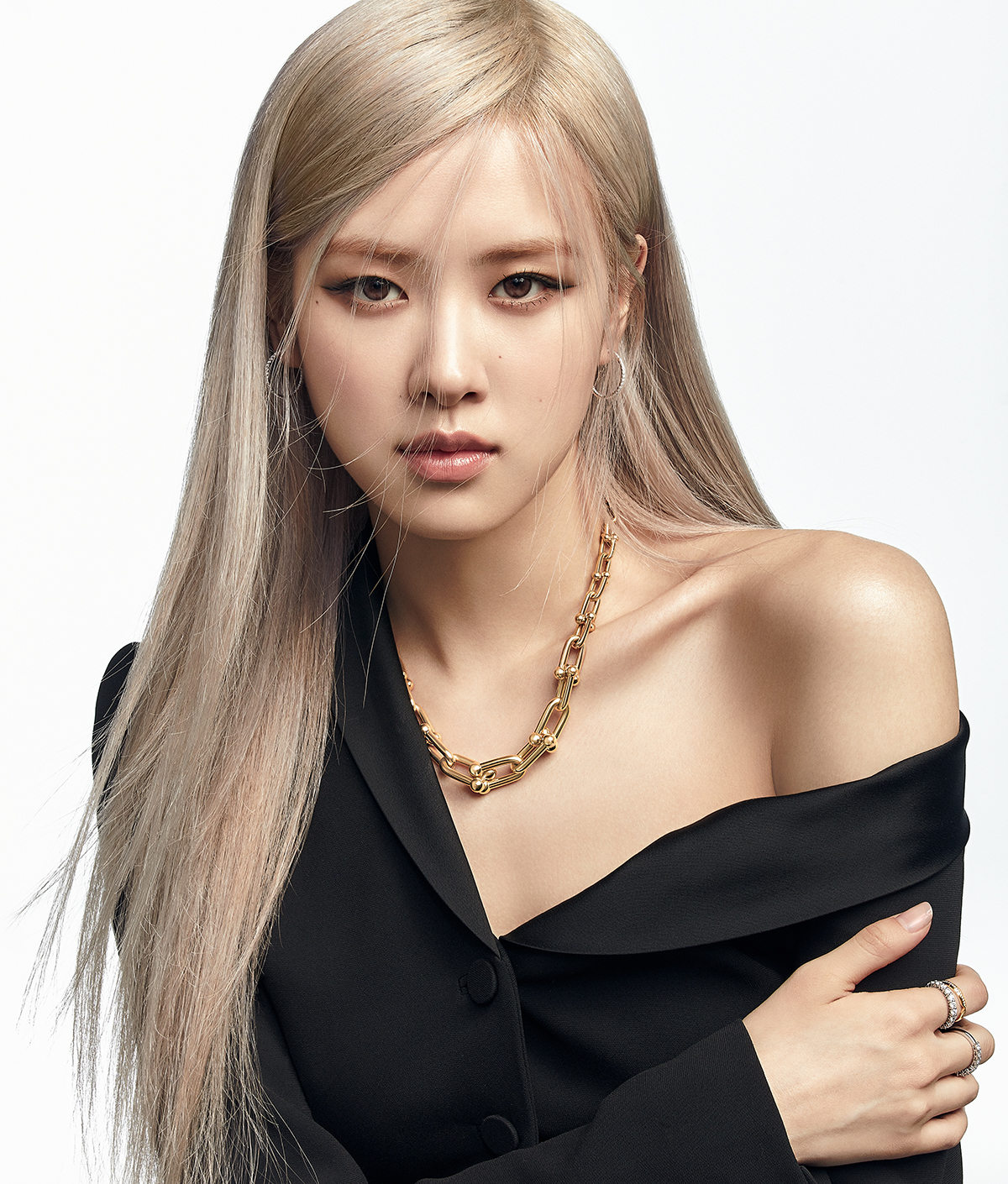
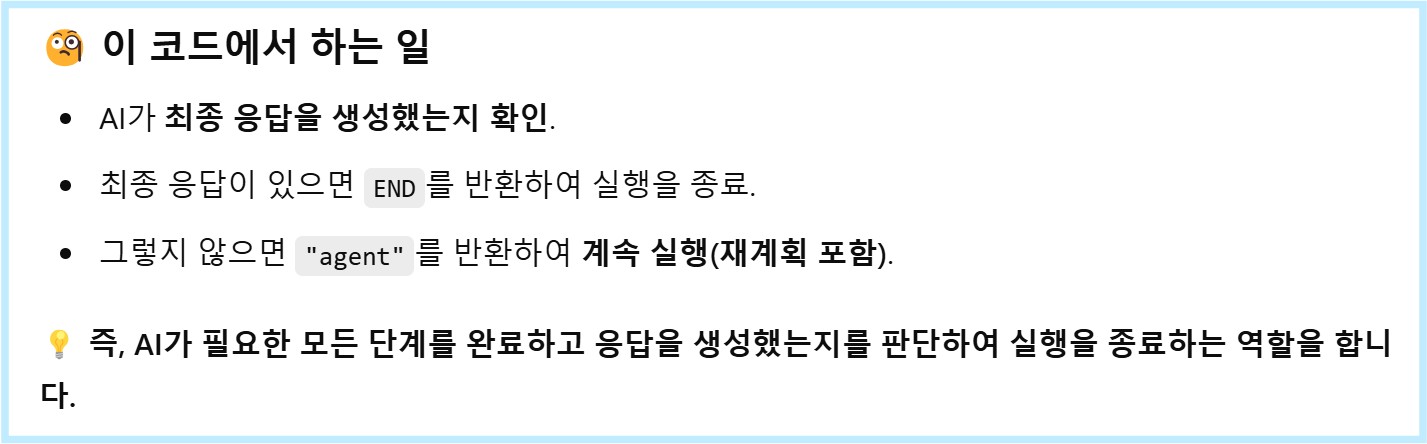
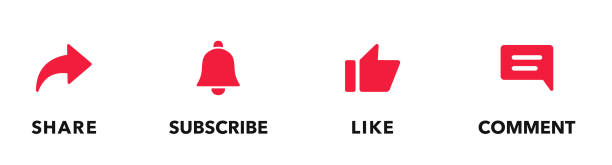
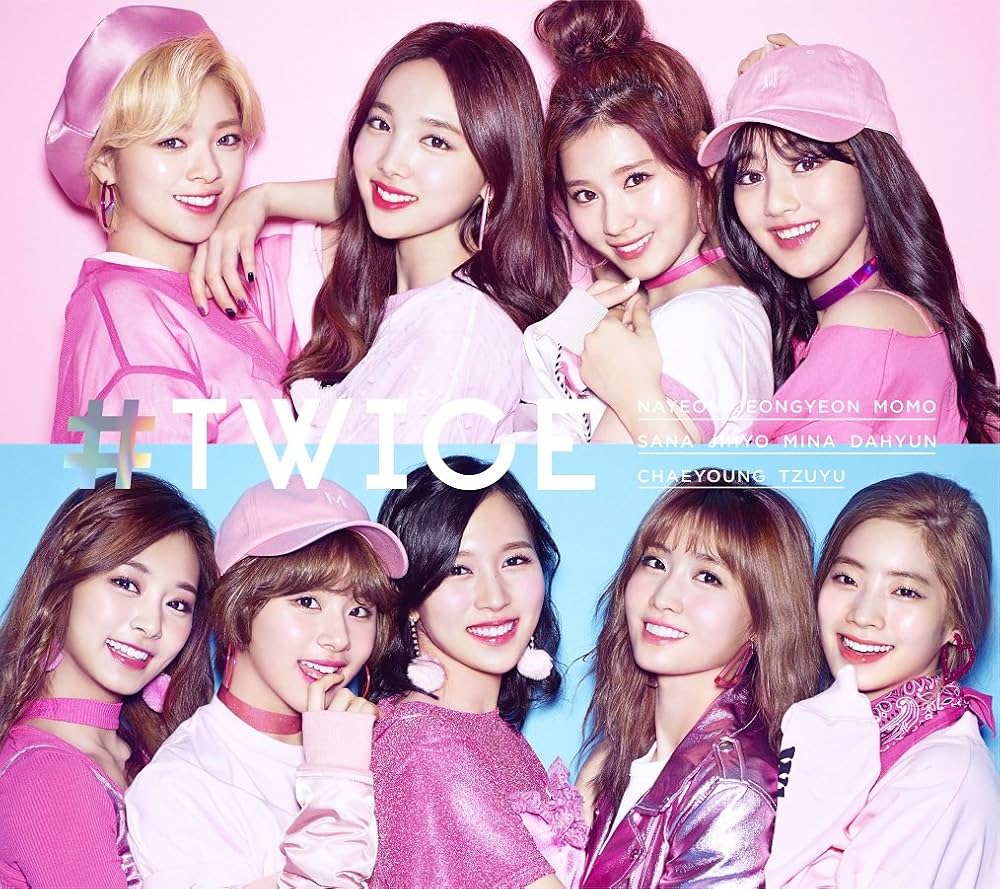
'Catchup AI' 카테고리의 다른 글
추론 방법론 비교 최종회 - 최강 AI 추론 모델들의 답변은? p.s. 일론 머스크 때문에 순위를 바꾼 사연 (0) | 2025.02.24 |
---|---|
같은 질문에 대해 AI 들은 얼마나 다르게 답변할까? - 추론 방법론을 물어 봤습니다. - (0) | 2025.02.22 |
AI 시대 준비는 추론에 대한 이해 부터... AI에게 질문을 잘하면 성공합니다. (0) | 2025.02.21 |
MS AI Agent 어플리케이션 개발 환경 Azure AI Foundry 를 쉽게 설명해 주네요. (0) | 2025.02.17 |
Microsoft Reactor 강좌 - Getting Started with Generative AI in Azure 1 (2) | 2025.02.16 |
AI Agent, Plan & Execute : Plan 편 - 가장 유명한 K-Pop Girl Group 멤버들의 생일은? 과연 AI 의 대답은? (1) | 2025.02.12 |
코딩의 판이 바뀐다 - Prompt를 지배하는자, AI Agent 개발의 챔피언이 됩니다. (0) | 2025.02.08 |
요즘 AI계의 핫 이슈인 추론에 대해 배웁니다 , Inference 와 Reasoning 의 차이점은? (0) | 2025.02.05 |
AI Agent hackathon 참가기 - 이렇게 AI에 미친들이 지금 미국을 바꾸고 있습니다. (0) | 2025.02.01 |
Prompt 중심으로 Backend 소스코드 분석 - AI와 기존 앱의 강점을 최대한 활용하는 것이 Agentic AI 입니다. (0) | 2025.01.31 |